The Journal of Physical Chemistry A, B, and C will publish a Virtual Special Issue (VSI) on machine learning in physical chemistry in 2020. The Guest Editors for the Virtual Special Issue Will Be: JPC A: Professor Thomas F. Miller III, California Institute of Technology JPC B: Associate Professor Andrew L. Ferguson, University of Chicago, and […]
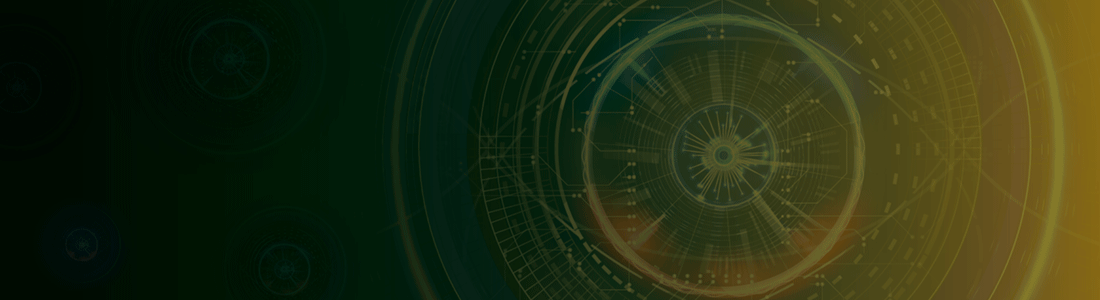
The Guest Editors for the Virtual Special Issue Will Be:
- JPC A: Professor Thomas F. Miller III, California Institute of Technology
- JPC B: Associate Professor Andrew L. Ferguson, University of Chicago, and Associate Professor Jim Pfaendtner, University of Washington
- JPC C: Assistant Professor Johannes Hachmann, University at Buffalo
Together they welcome researchers to submit their new and unpublished work by June 30, 2020.
If you are unsure if your research is within scope or have other questions about submitting a manuscript to this VSI, please email Editor-in-Chief Joan-Emma Shea’s office at shea-office@jpc.acs.org.