ACS In Focus recently held a virtual event on “Machine Learning in Chemistry: Now and in the Future” with Jon Paul Janet, Senior Scientist at AstraZeneca and co-author of the ACS In Focus Machine Learning in Chemistry e-book. This event had a brief discussion of Dr. Janet’s ACS In Focus e-book, a conversation on the […]
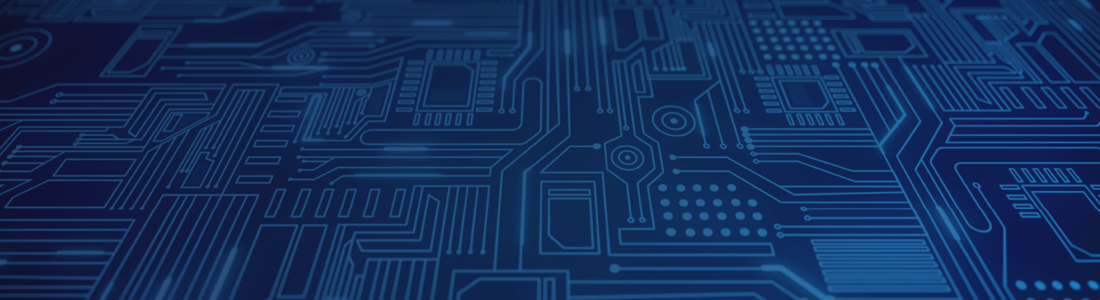
This event had a brief discussion of Dr. Janet’s ACS In Focus e-book, a conversation on the future of machine learning, and a presentation on the exciting research Dr. Janet and his colleagues have recently done using machine learning to accelerate the search for new materials.
Below you can watch the recording of the webinar and view some questions your colleagues asked.
View the Webinar Recording:
Interested in learning how to get access to Machine Learning in Chemistry? Talk to your librarian today!
Read Dr. Janet’s Answers to Community Questions
Definitely not! My Ph.D. group worked with a number of postdocs from purely chemistry backgrounds and there is a lot of domain experience that you gain from a Ph.D. that can be useful in applying machine learning methods. Probably I would recommend trying to join a group that does machine learning so you can learn from them. That said, being comfortable with python scripting (or some similar language) is pretty crucial and those skills take time to practice, so that might be a great additional skill to obtain. There are a lot of good online courses.
So we have been doing “machine learning in chemistry” for decades, the only difference now is that we have a larger toolbox of models that might or might not help build better activity models. QSAR methods in particular have benefited from a lot of optimization and seem to extract almost all the useful predictive power out of affinity data. If deep learning methods beat canonical QSAR approaches depends on who you ask, but in my experience, one is almost never worse off with ChemProp instead of a fingerprint method (though one might not be as much better off as one hopes). Sadly I don’t think we have gotten much better at activity prediction in the last few years, but neural networks also let us do interesting things in QSAR space such as large-scale multitask learning or even federated learning, and I think these approaches will be the standard in future. These methods give us a way to overcome the typically limited amount of affinity data we have for a particular target by bringing in more information. Some other limiting factors apart from dataset size are the quality of the data and the sensitivity to small structural changes (activity cliffs). These are pretty difficult to deal with since all machine learning models are smooth functions and struggle to learn large jumps (as humans sometimes do trying to rationalize SAR).