This Virtual Special Issue will highlight the use of machine learning in support of statistical mechanics-based methods for computation of thermophysical properties and phase equilibria. Submit your manuscript by December 31, 2023.
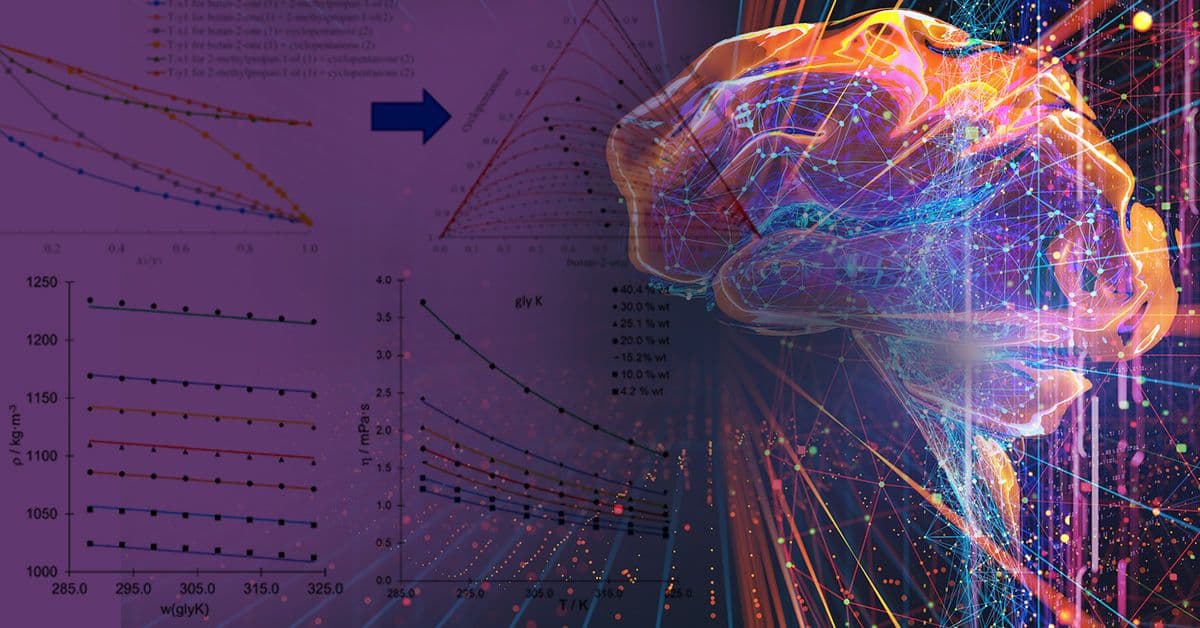
Machine learning (ML) is revolutionizing many fields, including the physical sciences. In the realm of property prediction, its obvious role is as a sophisticated means for correlating data, but often data are too scarce to permit this application. Instead, we can use ML to leverage methods rooted in statistical mechanics, and the Journal of Chemical & Engineering Data (JCED) is interested in publishing manuscripts that employ ML in this manner, rather than as a tool for correlation of properties. Examples include:
- Construction of potential energy surfaces via machine learning based on ab initio data, which are then used to compute properties by molecular simulation
- Using machine learning to accelerate or interpret MD or MC calculations
- Use of machine learning to supplement statistical mechanical theories for describing thermophysical properties
Application of big-data or machine-learning techniques to guide data collection and analysis in complex spaces, in conjunction with in-scope modeling methods or experimental measurements yielding new data
JCED is a monthly journal devoted to the publication of data obtained from both experiment and computation, which are viewed as complementary. It is the only ACS journal primarily concerned with articles containing data on the phase behavior and the physical, thermodynamic, and transport properties of well-defined materials, including complex mixtures of known compositions. JCED encourages manuscripts that report on consequential (relevant, comprehensive, and robust) data and place these data into context by addressing what can be learned from differences and similarities to prior published data on related systems.
The scope of this Journal also includes phase behavior and thermophysical properties obtained from quantum chemistry, molecular simulation, and molecular mechanics calculations. The reported data must be an observable property in these calculations via a statistical-mechanical approach. Manuscripts with a focus on computational approaches that predict thermophysical data via a correlation of molecular or other macroscopic properties do not generally fit within the scope of JCED. Also note that micellization, kinetics, and catalysis are not in scope.
Submission Instructions
Manuscripts must comply with the guidelines available on the JCED Information for Authors, General Guidelines for Authors, and Guidelines for Molecular Modeling and Simulation pages.
Submission must be made electronically through ACS Paragon Plus. In Paragon Plus, specify a manuscript type then select the Machine Learning for Thermophysical Properties Special Issue. In addition, state in your cover letter that the paper is being submitted for the Special Issue.
All invited and contributed manuscripts will be screened for suitability upon submission and undergo the standard peer-review procedure of the journal.
The manuscript submission deadline is December 31, 2023. For more information on the journal and manuscript submission, please visit the journal webpage. Queries can be sent to the editorial office at eic@jced.acs.org.
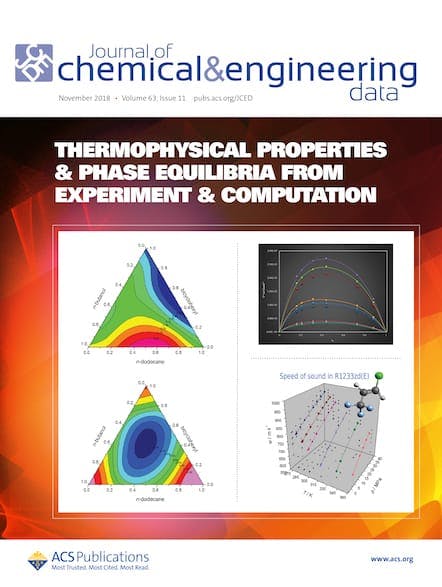