Machine learning is increasingly being used in research to process data and spot complex relationships. Here, we highlight several recent articles examining the pros and cons of using AI tools such as ChatGPT in environmental research.
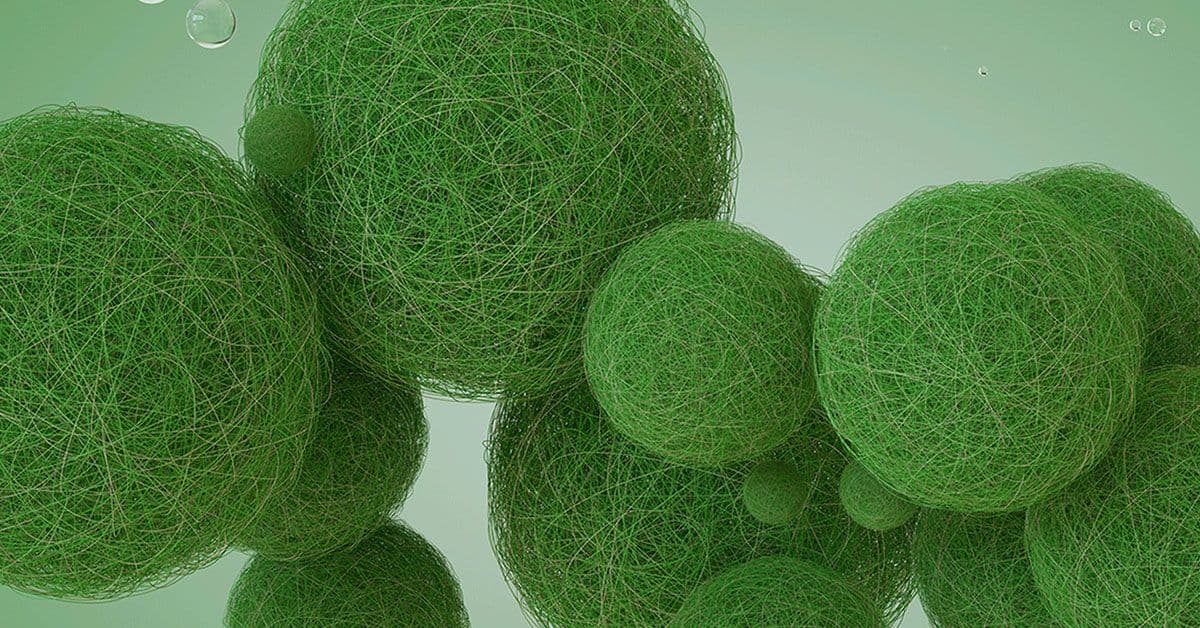
Artificial intelligence (AI) and tools such as ChatGPT are part of the machine learning revolution. Much has been said about them and the various shortcomings of their fledgling forms, but if we accept that these technologies are going to find their way into research, then we need to work out how best to harness them to achieve quality results.
Recently, a team of researchers from Princeton University published a review article in Environmental Science & Technology that critically examines both best practices and drawbacks of using AI and machine learning for environmental research. The authors point out the need to quickly understand and master the methodologies for harnessing these tools in research, as failure to do so may result in flawed work that could mislead other groups and compromise the validity of other studies. They present two reference paradigms for developing a supervised machine-learning model, inviting other researchers to follow, debate, improve, and customize these suggestions. The first lays out a four-step process for general supervised learning model development, from project set-up and data handling to model construction and applications. The second paradigm is more complex, covering a set of minimum requirements and best practices for these technologies specifically in environmental research.
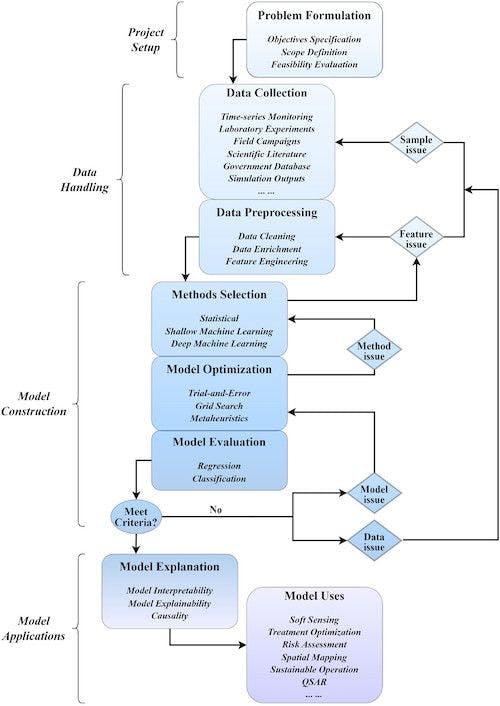
But if the quality of machine learning can be assured, then it can prove a powerful tool. ACS Sustainable Chemistry & Engineering recently published an article examining how a machine learning natural language processing (NLP) model could one day support plastic recycling. The researchers developed five different NLP tools—collectively named Recycle-BERT—to automatically extract, compile, and classify information from journals and databases relevant to a selected category of plastics, with the ultimate goal of establishing a circular economy of plastics recovery and recycling.
These five AI tools are designed to work together, each performing a slightly different task: Class-BERT classified the extracted literature as relevant or nonrelevant; Method-BERT scanned for recycling methods listed in the literature; Catalyst-BERT extracted catalyst details for recycling; Reactant-BERT found models to identify reactants; and Product-BERT pinpointed products formed from recycling. Upon testing the model, the results supported Recycle-BERT's ability to extract essential information from published literature, which could more easily help humans to make suitable recommendations for recycling practices.
Other researchers are optimistic that similarly positive outcomes could be seen in agriculture and water management, where AI could support sustainable and efficient systems as well as impact research. Within agriculture, such AI technologies could help to optimize farm practices and manage environmental challenges. Perhaps one of the most promising benefits, outlined in a recent Viewpoint article in ACS ES&T Water, is that by processing large amounts of scientific knowledge and communicating this information in a way that a broad audience can understand, tools such as ChatGPT can enable informed decisions at all levels—from individual households to global policy platforms, AI could help foster a sustainable, resilient future for water, agriculture, and the wider environment.
These perspectives also align with a recent review in ACS Nano that summarizes recent advances for AI applications in nanomaterials and nanotechnology, with emphasis on what this could mean for a net-zero emission future. The authors also discuss current limitations and challenges of AI in this area, identifying key gaps that will need to be addressed as well as future research opportunities to harvest AI’s full potential.
At present, the potential for AI's contributions to environmental and sustainability research is vast—and these tools themselves represent a pristine environment we've just begun exploring.