Many pharmaceutical drugs have side effects, but drug-induced cardiotoxicity represents a significant risk. A new study presents two possible frameworks that could offer a major leap in drug safety assessments.
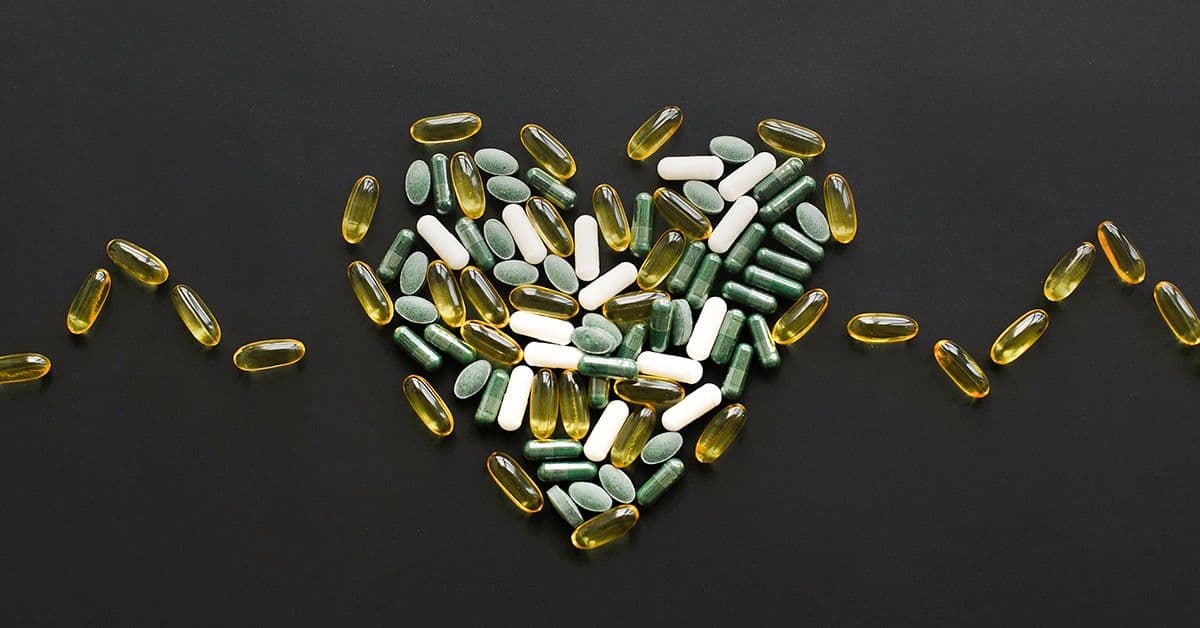
Many medications can inadvertently harm the heart, leading to serious conditions like irregular heartbeats (arrhythmias) or damage to heart muscle cells. Over the years, many pharmaceutical drugs have been reported to cause cardiovascular side effects, prompting their withdrawal from the market. It is critical to identify these risks early to protect patients and avoid costly recalls and wasted resources.
A new study published in ACS Sensors suggests that current methodologies—including traditional in vivo and in vitro models—have severe limitations when it comes to identifying cardiotoxic substances. To address these challenges, the authors developed a breakthrough approach using advanced artificial intelligence (AI) techniques to vastly improve the accuracy and reliability of drug safety assessments.
The researchers used lab-grown heart cells derived from induced pluripotent stem cells (iPSC-CMs), which more closely mimic human heart cells, and recorded mechanical beating signals through impedance measurements. These signals were then converted into enriched 2D representations through advanced transformation techniques and fed into the proposed frameworks. The team then analyzed the data using deep learning models, specifically short-time Fourier transform (STFT-CNN) and synchro-squeezing transform (SST-CNN), to classify drug types, determine drug concentrations, and identify cardiotoxic effects.
The framework based on STFT-CNN showed improvements over traditional methods, achieving an average accuracy of 93.8%. But the framework based on STT-CNN outperformed this, with 98.55% accuracy in drug type classification and 99% accuracy in distinguishing between cardiotoxic and non-cardiotoxic drugs.
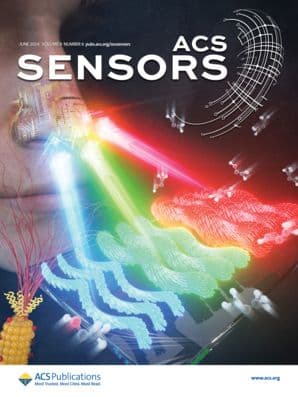
Two-Dimensional Deep Learning Frameworks for Drug-Induced Cardiotoxicity Detection
Similar work published in Journal of Chemical Information and Modeling has enabled prediction of very specific cardiac effects as well as general cardiotoxicity.2 An international guideline recommends all new drugs be assessed preclinically to see if they inhibit hERG, a gene that plays a crucial role in the cardiac myocyte.3 This is because hERG blockade results in prolongation of the QT interval, and ultimately fatal cardiotoxicity. The study used a deep neural network, with the algorithm achieving a satisfactory predictive ability.
Deep learning has also been used to predict drug-induced liver injury—the single most frequent cause of safety-related drug marketing withdrawals for the past 50 years.4 Critically, agents that cause severe liver injury in humans typically do not show clear hepatotoxicity in animals, which limits the use of traditional preclinical toxicology studies. But deep-learning models have shown accuracy of 86.9%, sensitivity of 82.5%, and specificity of 92.9%, as well as allowing identification of important molecular features. A more recent integrated artificial intelligence and machine learning model has been shown to be able to predict liver-injury severity for small-molecule agents using a combination of physicochemical properties and off-target interactions predicted in silico, improving predictions compared to when using only chemical properties.5
The drug development process faces a high failure rate, with around 90% of drugs failing to pass clinical trials.6 With the potential for scalability, these new deep-learning approaches represent a major leap forward in performing drug safety assessments, and offer a way to achieve more robust and efficient toxicity evaluations that are directly relevant to humans.
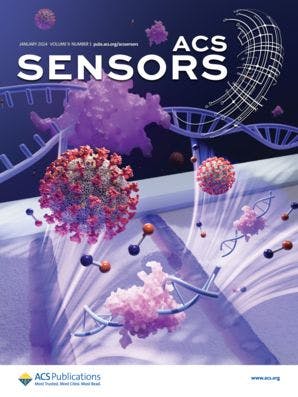