The Journal of Medicinal Chemistry is planning to publish a Special Issue titled “Artificial Intelligence in Drug Discovery” in June 2020, headed up by Guest Editors Professor Jürgen Bajorath, University of Bonn and University of Washington, Dr. Steven Kearnes, Google Research, Applied Science Team, and Dr. W. Patrick Walters, Relay Therapeutics. The Special Issue aims […]
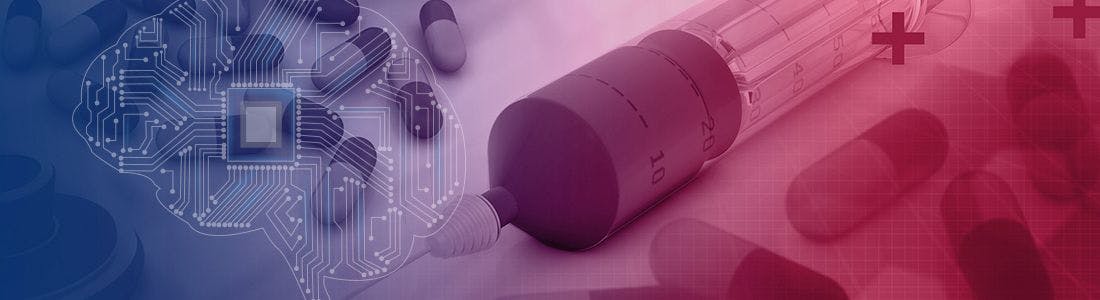
The Journal of Medicinal Chemistry is planning to publish a Special Issue titled “Artificial Intelligence in Drug Discovery” in June 2020, headed up by Guest Editors Professor Jürgen Bajorath, University of Bonn and University of Washington, Dr. Steven Kearnes, Google Research, Applied Science Team, and Dr. W. Patrick Walters, Relay Therapeutics.
The Special Issue aims to highlight the emerging role of AI, including machine learning and other approaches such as expert systems and generative models in early phase drug discovery. The Editors will consider a wide range of contributions for the Special Issue, including off-the-beaten-path concepts, methods, and applications.
If you’re not sure if your research is within the issue’s scope, please send inquiries to bajorath-office@jmedchem.acs.org for feedback, or read the editorial for more information.
Insights from Guest Editors
I asked Guest Editors Pat Walters and Steve Kearnes, to provide insight on what kind of papers they are looking for in this Special Issue, and what excites them about artificial intelligence and drug discovery.
What are the most exciting innovations and discoveries in artificial intelligence and drug discovery that you’ve read about recently?
“There are three applications of machine learning in drug discovery that excite me:
- Applications of deep learning to the identification of specific cellular phenotypes. A recent paper in the Lancet showed that even individuals with no programming experience were able to use an “off the shelf” machine learning framework to create reliable workflows for image classification.
- Machine learning of quantum chemical potentials. Work by Roitberg and others enables calculations that required days using traditional methods to be carried out in less than a second.
- Applications of deep learning to organic synthesis planning. A number of recent papers have demonstrated that deep learning can be used to develop plausible synthetic routes for complex molecules,” said Walters.
“Many of the applications of machine learning to drug discovery have been limited by the available data, and I’m excited to see a trend toward gathering data that is better suited for training deep learning models. One aspect of this is a focus on molecular properties that are common to many programs, such as ADME/Tox. Another approach is “active learning,” where data is generated iteratively to improve the model in regions of uncertainty,” said Kearnes.
Anything else you’d like to tell people about the Special Issue?
“I would love to see examples where the value of a machine learning model in discovery can be quantified and compared to other approaches. Most experiments involve subjective filtering of model predictions that make this very difficult to measure,” said Kearnes.
Walters added, “First and foremost, I would like to see practical applications of AI that have influenced the direction of drug discovery projects.”
What to Submit—Deadline: December 31, 2019
Articles, Brief Articles, Drug Annotations, and Perspectives are all welcome. For details on requirements for each manuscript type, please consult the Author Guidelines. You can also find the Journal of Medicinal Chemistry scope below.
Journal of Medicinal Chemistry Scope
The Journal of Medicinal Chemistry publishes studies that contribute to an understanding of the relationship between molecular structure and biological activity or mode of action.
Some appropriate, specific areas include the following:
- Design, synthesis, and biological evaluation of novel biologically active compounds, diagnostic agents, or labeled ligands employed as pharmacological tools.
- Molecular modifications of reported series that lead to a significantly improved understanding of their structure-activity relationships (SAR). Routine extensions of existing series that do not utilize novel chemical or biological approaches or do not add significantly to a basic understanding of the SAR of the series will normally not be accepted for publication.
- Structural biological studies (X-ray, NMR, etc.) of relevant ligands and targets with the aim of investigating molecular recognition processes in the action of biologically active compounds.
- Molecular biological studies (e.g., site-directed mutagenesis) of macromolecular targets that lead to an improved understanding of molecular recognition.
- Computational studies that provide fresh insight into the SAR of compound series that are of current general interest or analysis of other available data that subsequently advance medicinal chemistry knowledge.
- Substantially novel computational chemistry methods with demonstrated value for the identification, optimization, or target interaction analysis of bioactive molecules.
- Effect of molecular structure on the distribution, pharmacokinetics, and metabolic transformation of biologically active compounds. This may include design, synthesis, and evaluation of novel types of prodrugs.
- Novel methodology with broad application to medicinal chemistry, but only if the methods have been tested on relevant molecules.