Artificial intelligence and machine learning are incredibly powerful tools with the potential to fundamentally change research and data processing methods—but can they change the way we eat and grow food?
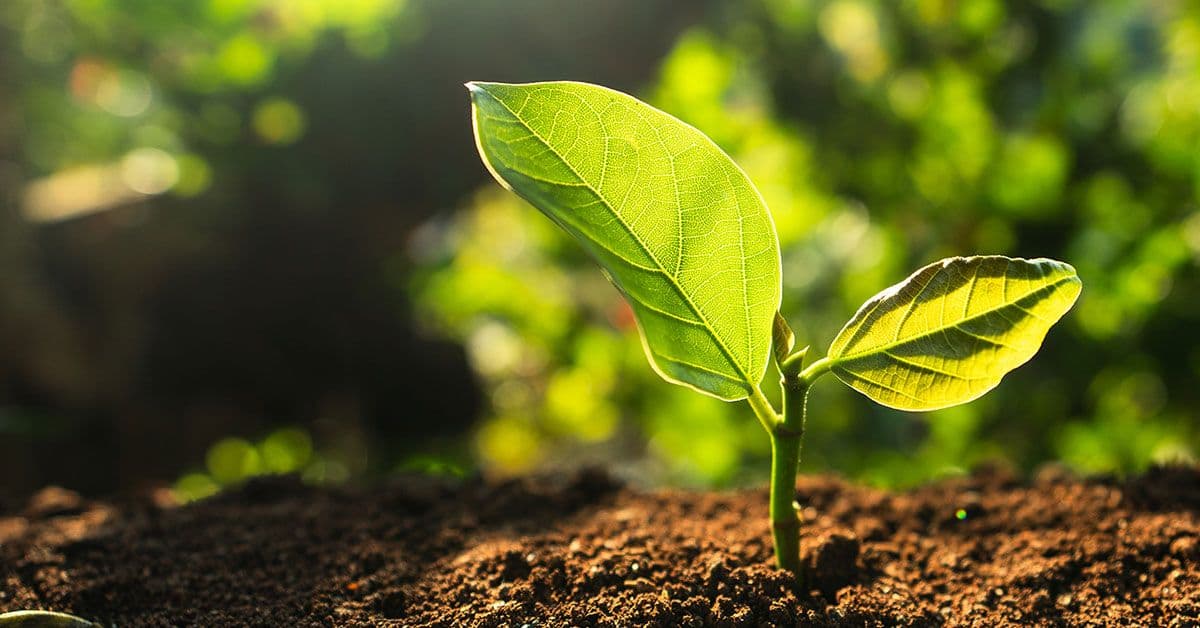
Artificial intelligence (AI) and machine learning (ML) are becoming increasingly ubiquitous across numerous industries and fields, and the chemical sciences are no exception. These incredibly powerful tools are fundamentally changing the way we learn and process data, with huge implications both academically and commercially. This disruption is also being felt within the fields of agricultural and food chemistry, where AI and ML could provide solutions and fill common knowledge gaps for natural crops, processed foods, storage solutions, and much more. Read on to discover some of the latest research showcasing the potential of AI and ML in helping shape the future of food science.
Extending Shelf Life
Take the avocado—a great source of healthy fats, and the perfect choice for breakfast. Avocados have rapidly evolved from trend to staple across restaurant menus worldwide, and global avocado production has almost quadrupled in the last 20 years. However, the limited shelf life of an avocado remains a challenge, and it is difficult to monitor without opening and often wasting test fruits. New research published in ACS Food Science & Technology aims to change that, with the help of machine learning. By using hyperspectral imaging (HSI) across three dimensions— two spatial and one spectral—in combination with ML, researchers were able to successfully estimate the impact of protective chitosan coatings on the quality and shelf life of avocados across various key quality indicators.
For each property, several artificial neural networks were tested for efficacy. In general, Bayesian regularization neural networks outperformed other ML techniques in estimating quality attributes from the imaging features. This method demonstrates the potential for HSI and ML to be effectively used together to provide non-invasive, real-time assessments of food quality without having to waste sample produce.
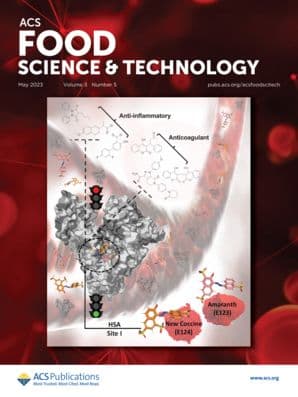
Postharvest Treatments of Hass Avocado (Persea americana Mill.) and Estimation of Its Quality Using Hyperspectral Imaging (HSI)
ACS Food Sci. Technol. 2023, 3, 5, 932–944
Get full access to this journal.
Shelf life is also a challenge for food products that contain unsaturated fatty acids, which are prone to lipid oxidation and can easily develop a rancid odor and flavor. Traditionally, the solution has been to add antioxidants, but certain combinations can have adverse effects—and empiric research has been unable to account for their multifaceted responses. To address this knowledge gap, a team in the United States and Argentina worked together on an AI deep-learning architecture to predict antioxidant interactions, publishing their findings in the Journal of Agricultural and Food Chemistry.
At first, the results were disappointing, and the AI struggled with predicting how well certain antioxidant mixtures would behave in actual product samples. But after the authors added additional data from prior experiments to the AI model, the algorithm became more reliable in figuring out how well antioxidant mixtures would work in preventing the negative effects of lipid oxidation. This study has tremendous value far beyond antioxidant applications—a key takeaway is that AI is only as helpful as the information it knows, and providing AI tools with comprehensive and chemically relevant experimental data is crucial for the technology to act as a reliable assistant in scientific research.
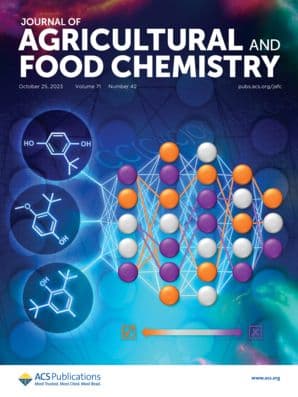
Predicting Antioxidant Synergism via Artificial Intelligence and Benchtop Data
J. Agric. Food Chem. 2023, 71, 42, 15644–15655
Get full access to this journal.
Detecting Foodborne Contaminants
AI has also demonstrated potential in detecting foodborne contaminants. The Journal of Agricultural and Food Chemistry recently published novel experimental research using ML to detect mycotoxins from phytopathogenic fungi, which often find their way into food during production and storage and can have harmful effects on humans and animals when consumed.
The authors revealed a first-of-its-kind integrated approach using carbon dots and ML algorithms to simultaneously detect three common mycotoxins in samples of coffee, milk, and several grains with 100% accuracy. The authors note that this methodology demonstrates the tremendous potential for AI and ML to help improve the accuracy and efficiency of foodborne contaminant detection.
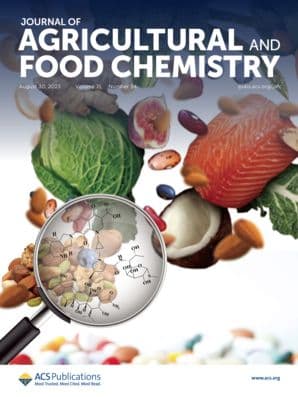
Machine Learning-Mediated Ultrasensitive Detection of Citrinin and Associated Mycotoxins in Real Food Samples Discerned from a Photoluminescent Carbon Dot Barcode Array
J. Agric. Food Chem. 2023, 71, 34, 12849–12858
Get full access to this journal.
Controlling Crop Disease
Huanglongbing (HLB), also known as citrus greening disease, is one of the most devastating diseases for citrus plants, causing them to wither and die within a few years and leading to substantial economic losses. There is currently no known cure; however, a team working in Florida, United States, hypothesized that citrus plant microbiomes may serve as disease antagonists, increasing the level of tolerance for HLB. By inhibiting pathogens directly or triggering the plant’s immune response, these microbiomes can act almost as an extended immune system for the plants.
To investigate this, the researchers introduced a novel multiomics approach, combining metabolomics (studying metabolites) and gene sequencing (studying bacteria) using machine learning techniques. Their results demonstrate that regulation of plant pathways could affect the structure of the microbial community, hinting at potential solutions for controlling this economically devastating disease by modulating bacteria in citrus plants—or breeding tolerant cultivars. This study reinforces the utility of ML in multiomics due to its ability to uncover new or hidden information from experimental data and analytical methods.
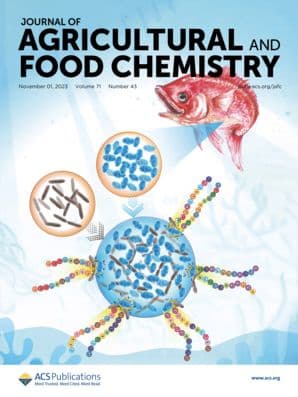
Uncovering Interactions between Plant Metabolism and Plant-Associated Bacteria in Huanglongbing-Affected Citrus Cultivars Using Multiomics Analysis and Machine Learning
J. Agric. Food Chem. 2023, 71, 43, 16391–16401
Get full access to this journal.
Gaining Insight Into Flavor Perception
We’ve covered how AI and ML can be used to help improve food composition and freshness, supply chain management, and crop health, but could these tools also uncover a deeper understanding of our sensory response to aroma and taste? Many foods for humans play a role far beyond simple nutrition, but taste is a subjective concept and sensory evaluations are difficult to measure. The five basic tastes are sweet, sour, bitter, salty—and the elusive umami, which in its native Japanese translates as “tasty” or “delicious” and describes deeply savory and satisfying flavors. Many describe foods such as mushrooms, tomatoes, aged cheeses, meats, and soy sauce (among others) as having umami flavors.
Despite the subjectivity of perceived flavor profiles, we do know that the umami taste is mediated by G-protein coupled receptors and associated with specific signals to taste receptor cells. With this information, a team in China used electroencephalography and machine learning to identify umami in foods by recording electrical waves during brain activity. To characterize brain activity when presented with umami taste stimuli, the researchers employed a model that uses 125 brain wave signals across five regions as input data. The model was then published online, making it accessible for other researchers to use for further studies. The authors note that this model could have applications in diagnosing diseases through taste dysfunction and may lead to future generations of brain–computer interfaces for flavor perception.
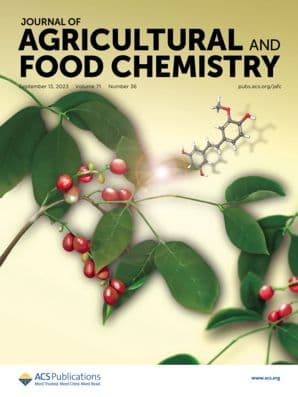
TastePeptides-EEG: An Ensemble Model for Umami Taste Evaluation Based on Electroencephalogram and Machine Learning
J. Agric. Food Chem. 2023, 71, 36, 13430–13439
Get full access to this journal.
Get Access to This Research and More
Watch the video below to find out how ACS Publications cross-disciplinary resources can help drive discovery in your own research.