A new study reveals how advanced machine learning and spectroscopic techniques can be used together to transform the analysis and preservation of modern artwork.
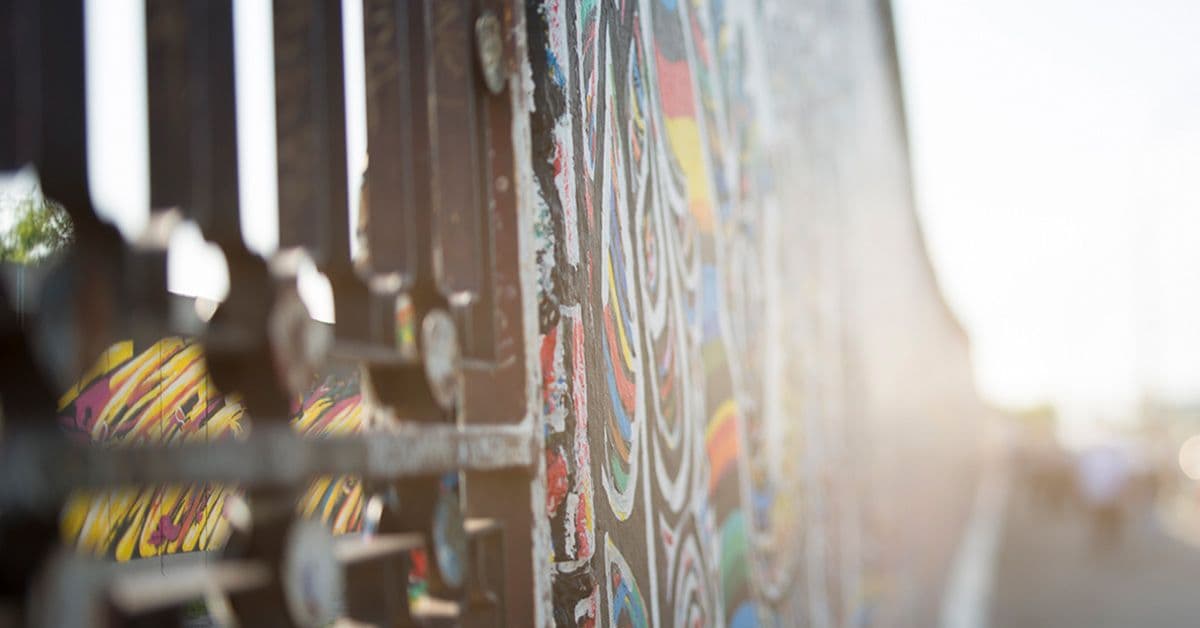
Understanding how a piece of art has been created is important—both for art historians and critics, but also for conservationists and restorers, who must take great care to ensure that any cleaning or repair is sympathetic to the original materials and will not cause any unforeseen reactions with chemicals in the paint or substrate. We looked previously at how da Vinci used different materials in a thick layer between the wooden panel and the final image, but understanding artists' materials and techniques doesn’t apply only to the Old Masters. Many modern works also need preservation, especially those that have been created as part of our street scenes or in the course of significant cultural events. A notable example is the vibrant murals made on the Berlin Wall. This famous barrier stood from 1961 until 1989, separating East and West Berlin—and the very different political situations in the two halves of Europe after the Second World War. Both before and after its fall, artists used the Wall as a canvas for both political and artistic expression.
Today, the remains are described as the world’s longest open-air gallery,1 but being completely exposed to the weather, there are regular efforts to restore and maintain the paintings. Yet even with these works made so recently, most artists left their mark anonymously and therefore didn't document their materials or techniques. Furthermore, while there are very comprehensive databases of chemical characterization for ancient inorganic and organic pigments, information remains limited for more modern dyes and paints.
Now, new research published in the Journal of the American Chemical Society focuses on analyzing 15 fragments of five different colors from Berlin Wall street art to better identify and classify the materials and techniques the artists used.2 Using multispectral photographic recognition, the researchers determined that all of the fragments came from 2-3 layers of acrylic paint, applied with a brush. Then, using handheld Raman spectroscopy devices, the team identified the primary pigments as azopigments, phthalocyanins, lead chromate, and titanium white.
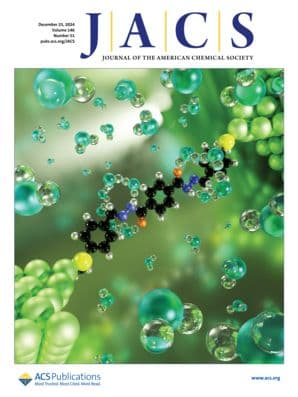
Chemistry of Street Art: Neural Network for the Spectral Analysis of Berlin Wall Colors
To further their research, the team mixed commercial acrylic paints with different ratios of titanium white to match the colors used by the original artists. They then developed and trained an AI algorithm to analyze the spectral data from these mixtures and predict the ratio between coloring compounds. The authors note that this novel AI model could provide valuable information for art conservation and offer new opportunities for analytical quantification using hand-held spectrometers.
Further Explorations: Browse Additional Research from ACS Journals
This is not the first time spectroscopic analysis and machine learning have been used to better understand materials in art, literature, and beyond. Explore additional articles highlighting these topics and techniques from ACS journals:
Identification of Synthetic Organic Pigments (SOPs) Used in Modern Artist’s Paints with Secondary Ion Mass Spectrometry with MeV Ions
Matea Krmpotić*, Dubravka Jembrih-Simbürger, Zdravko Siketić, Nikola Marković, Marta Anghelone, Tonči Tadić, Dora Plavčić, Mason Malloy, and Iva Bogdanović Radović
DOI: 10.1021/acs.analchem.0c01630
A Machine Learning Protocol for Predicting Protein Infrared Spectra
Sheng Ye, Kai Zhong, Jinxiao Zhang, Wei Hu, Jonathan D. Hirst, Guozhen Zhang, Shaul Mukamel, and Jun Jiang*
DOI: 10.1021/jacs.0c06530
Near-Infrared Spectroscopy and Machine Learning for Accurate Dating of Historical Books
Floriana Coppola*, Luca Frigau, Jernej Markelj, Jasna Malešič, Claudio Conversano, and Matija Strlič
DOI: 10.1021/jacs.3c02835
Uncovering Art’s Vanishing Hues with Surface-Enhanced Raman Scattering: Drawing Inspiration from the Past for the Future
Kristin L. Wustholz*, Shelley A. Svoboda, Meredith G. Martin, Benjamin T. Steinman, and Zhaoyun Zheng
DOI: 10.1021/acsnano.4c05389
Machine Learning-Assisted Surface-Enhanced Raman Spectroscopy Detection for Environmental Applications: A Review
Sonali Srivastava, Wei Wang, Wei Zhou, Ming Jin, and Peter J. Vikesland*
DOI: 10.1021/acs.est.4c06737
References
- https://www.visitberlin.de/en/east-side-gallery
- Armetta, F. et al. Chemistry of Street Art: Neural Network for the Spectral Analysis of Berlin Wall Colors. J. Am. Chem. Soc. 2024, 146, 51, 35321–35328.