The 2024 Nobel Prize in Chemistry was awarded to David Baker “for computational protein design,” and Demis Hassabis and John Jumper “for protein structure prediction.” Browse noteworthy articles surrounding the winning research in ACS journals.
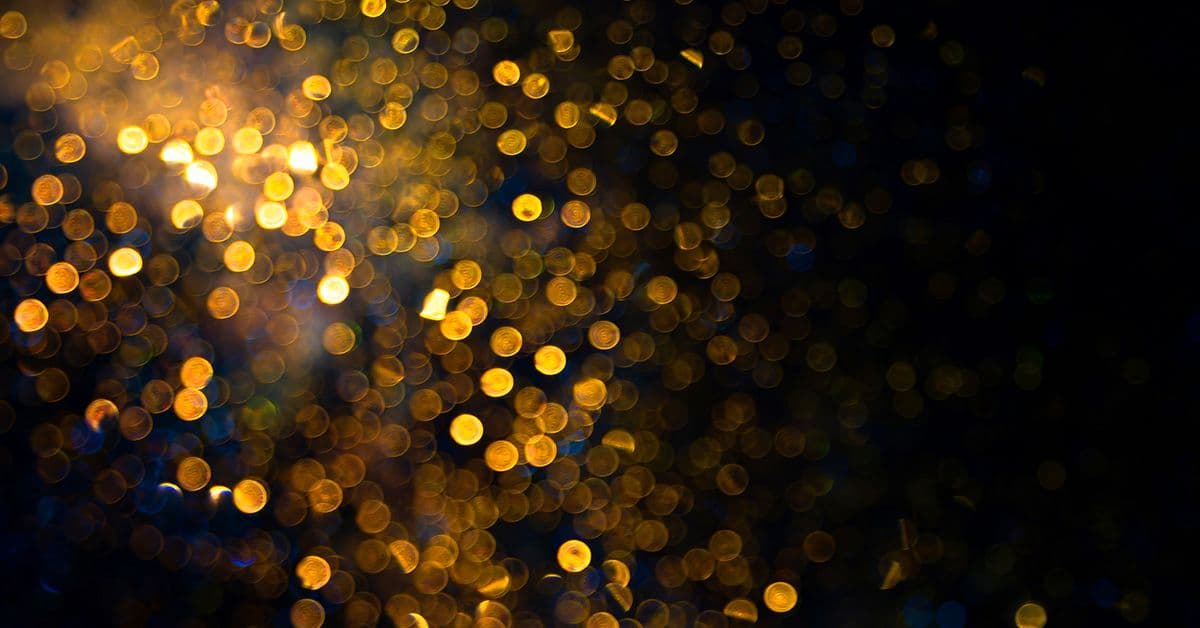
The 2024 Nobel Prize in Chemistry was awarded with one half to David Baker “for computational protein design,” and the other half jointly to Demis Hassabis and John Jumper “for protein structure prediction.”
“This incredibly complex problem of predicting the 3D structures of proteins from the sequence of amino acids has been one of the biggest challenges in chemistry. We care about this because structure determines function — that’s one of the fundamental tenets of chemistry. The ability to design new proteins goes hand in hand with predicting the structure of proteins,” says ACS President Mary K. Carroll. “The wonderful complexity of proteins has made these formidable tasks, and the solutions have relied on the power of neural networks and sophisticated computational work combined with fundamental knowledge of the underlying chemistry.”
Baker is the winner of the 2014 David Perlman Memorial Award, presented by ACS’ Division of Biochemical Technology. His talk on “The Coming of Age of De Novo Protein Design” is featured in the ACS Publications webinar “Grand Challenges in Bio & Medicinal Chemistry Frontiers in Carbohydrate and Protein Chemistry,” which is available to watch on demand. Baker gave a plenary talk on “Post-Evolutionary Biology: Design of Novel Protein Structures, Functions and Assemblies” at ACS Spring 2016.
Jumper is part of the ACS member community and gave a plenary talk on “Expanding the Role of Machine Learning in Chemistry” at ACS Fall 2023.
Notably, Baker has published extensively in some of ACS’ more than 85 peer-reviewed journals throughout his career. The following selection of articles by Baker, as well as related articles published in ACS journals, are available free-to-read through the end of October 2024.
A Closer Look: Protein Design and Structure
Proteins are long molecules made up of amino acid residues strung together. Short proteins might be less than a hundred such residues, while large proteins contain tens of thousands. These long molecular chains “fold” themselves into three-dimensional structures, and this folding determines what the protein does.
A long-standing challenge in protein chemistry is predicting this structure, and hence the protein function, from the sequence of amino acids that goes into it. Since proteins serve such a wide range of functions in every organism, and are the mechanism by which our DNA expresses itself, connecting sequence to function would allow for new medicines and is a requirement for effective gene editing.
A huge amount of work has gone into this protein structure prediction problem, and any number of theoretical approaches were tried. David Baker and colleagues made an enormous step forward by realizing that we don’t have to understand the physical basis of the many interactions that protein segments undergo with each other to drive structure formation, but instead could use the many thousands of existing structures to identify peptide sequences that would create a desired piece of protein structure—which could then be assembled into larger pieces with small adjustments.
The result was Rosetta, a program to design proteins for specific structures (and hence functions), which became the standard for researchers across the protein engineering community.
In one of the biggest surprises in all of science, the next solution to the protein structure prediction problem didn’t come from a new theory of biochemistry—it came from a collision with the advancing field of machine learning.
Researchers at DeepMind realized that this same huge body of carefully identified protein structures—decades' worth of work from researchers all over the world—could be fed into a machine learning algorithm which could then predict new structures simply from the patterns it had picked up on by observing nature.
The results shocked everyone. In ten years, this machine learning approach went from an interesting idea to the most accurate algorithm available, leaping over the established approaches and becoming so accurate that the problem of protein structure is considered by many to be solved—a solution that involved no new theory or increased computer power, simply the right program to pull out the patterns that were hidden in nature all along. It was a stunning display of the power of machine learning to tackle the biggest problems in science, and it created a new tool of which we’ve barely begun to tap the potential.
The two approaches work hand-in-hand: DeepMind predicting structure and function from an amino acid sequence, and the Rosetta providing an amino acid sequence to fit a given structure and function. With these programs, researchers will be able to understand our genes and how they interact as never before, create enzymes to attack cancer cells or pull carbon out of the atmosphere, or create new food sources. Most exciting of all are the uses we haven’t even thought of yet for these new techniques in understanding and creating protein structures.
Explore ACS Journal Articles by David Baker
Enumerative Discovery of Noncanonical Polypeptide Secondary Structures
Adam P. Moyer, Theresa A. Ramelot, Mariano Curti, Margaret A. Eastman, Alex Kang, Asim K. Bera, Roberto Tejero, Patrick J. Salveson, Carles Curutchet, Elisabet Romero, Gaetano T. Montelione*, and David Baker*
J. Am. Chem. Soc. 2024, 146, 37, 25501–25512
DOI: 10.1021/jacs.4c04991
Computational Design of Cyclic Peptide Inhibitors of a Bacterial Membrane Lipoprotein Peptidase
Timothy W. Craven, Mark D. Nolan, Jonathan Bailey, Samir Olatunji, Samantha J. Bann, Katherine Bowen, Nikita Ostrovitsa, Thaina M. Da Costa, Ross D. Ballantine, Dietmar Weichert, Paul M. Levine, Lance J. Stewart, Gaurav Bhardwaj, Joan A. Geoghegan*, Stephen A. Cochrane*, Eoin M. Scanlan*, Martin Caffrey*, and David Baker*
ACS Chem. Biol. 2024, 19, 5, 1125–1130
DOI: 10.1021/acschembio.4c00076
Design of Diverse Asymmetric Pockets in De Novo Homo-oligomeric Proteins
Stacey R Gerben, Andrew J Borst, Derrick R Hicks, Isabelle Moczygemba, David Feldman, Brian Coventry, Wei Yang, Asim K. Bera, Marcos Miranda, Alex Kang, Hannah Nguyen, and David Baker*
Biochemistry 2023, 62, 2, 358368
DOI: 10.1021/acs.biochem.2c00497
Increasing Computational Protein Design Literacy through Cohort-Based Learning for Undergraduate Students
Erin C. Yang, Robby Divine, Christine S. Kang, Sidney Chan, Elijah Arenas, Zoe Subol, Peter Tinker, Hayden Manninen, Alicia Feichtenbiner, Talal Mustafa, Julia Hallowell, Isiac Orr, Hugh Haddox, Brian Koepnick, Jacob O’Connor, Ian C. Haydon, Karla-Luise Herpoldt, Kandise Van Wormer, Celine Abell, David Baker, Alena Khmelinskaia*, and Neil P. King*
J. Chem. Educ. 2022, 99, 9, 3177–3186
DOI: 10.1021/acs.jchemed.2c00500
The Rosetta All-Atom Energy Function for Macromolecular Modeling and Design
Rebecca F. Alford, Andrew Leaver-Fay, Jeliazko R. Jeliazkov, Matthew J. O’Meara, Frank P. DiMaio, Hahnbeom Park, Maxim V. Shapovalov, P. Douglas Renfrew, Vikram K. Mulligan, Kalli Kappel, Jason W. Labonte, Michael S. Pacella, Richard Bonneau, Philip Bradley, Roland L. Dunbrack Jr., , Rhiju Das, David Baker, Brian Kuhlman, Tanja Kortemme, and Jeffrey J. Gray*
J. Chem. Theory Comput. 2017, 13, 6, 3031–3048
DOI: 10.1021/acs.jctc.7b00125
Engineering of Kuma030: A Gliadin Peptidase That Rapidly Degrades Immunogenic Gliadin Peptides in Gastric Conditions
Clancey Wolf, Justin B. Siegel, Christine Tinberg, Alessandra Camarca, Carmen Gianfrani, Shirley Paski, Rongjin Guan, Gaetano Montelione, David Baker, and Ingrid S. Pultz*
J. Am. Chem. Soc. 2015, 137, 40, 13106–13113
DOI: 10.1021/jacs.5b08325
Computational Design of an Unnatural Amino Acid Dependent Metalloprotein with Atomic Level Accuracy
Jeremy H. Mills, Sagar D. Khare, Jill M. Bolduc, Farhad Forouhar, Vikram Khipple Mulligan, Scott Lew, Jayaraman Seetharaman, Liang Tong, Barry L. Stoddard, and David Baker*
J. Am. Chem. Soc. 2013, 135, 36, 13393–13399
DOI: 10.1021/ja403503m
Computational Design of Enone-Binding Proteins with Catalytic Activity for the Morita–Baylis–Hillman Reaction
Sinisa Bjelic, Lucas G. Nivón, Nihan Çelebi-Ölçüm, Gert Kiss, Carolyn F. Rosewall, Helena M. Lovick, Erica L. Ingalls, Jasmine Lynn Gallaher, Jayaraman Seetharaman, Scott Lew, Gaetano Thomas Montelione, John Francis Hunt, Forrest Edwin Michael, K. N. Houk, and David Baker*
ACS Chem. Biol. 2013, 8, 4, 749–757
DOI: 10.1021/cb3006227
Protein NMR Structures Refined with Rosetta Have Higher Accuracy Relative to Corresponding X-ray Crystal Structures
Binchen Mao, Roberto Tejero, David Baker, and Gaetano T. Montelione*
J. Am. Chem. Soc. 2014, 136, 5, 1893–1906
DOI: 10.1021/ja409845w
Improving the Catalytic Performance of an Artificial Metalloenzyme by Computational Design
Tillmann Heinisch, Michela Pellizzoni, Marc Dürrenberger, Christine E. Tinberg, Valentin Köhler, Juliane Klehr, Daniel Häussinger, David Baker*, and Thomas R. Ward*
J. Am. Chem. Soc. 2015, 137, 32, 10414–10419
DOI: 10.1021/jacs.5b06622
Related ACS Journal Articles
AlphaFold 2: Why It Works and Its Implications for Understanding the Relationships of Protein Sequence, Structure, and Function
Jeffrey Skolnick*, Mu Gao, Hongyi Zhou, and Suresh Singh
J. Chem. Inf. Model. 2021, 61, 10, 4827–4831
DOI: 10.1021/acs.jcim.1c01114
Recent Advances and Challenges in Protein Structure Prediction
Chun-Xiang Peng, Fang Liang, Yu-Hao Xia, Kai-Long Zhao, Ming-Hua Hou, and Gui-Jun Zhang*
J. Chem. Inf. Model. 2024, 64, 1, 76–95
DOI: 10.1021/acs.jcim.3c01324
A Perspective on the Prospective Use of AI in Protein Structure Prediction
Raphaelle Versini, Sujith Sritharan, Burcu Aykac Fas, Thibault Tubiana, Sana Zineb Aimeur, Julien Henri, Marie Erard, Oliver Nüsse, Jessica Andreani, Marc Baaden, Patrick Fuchs, Tatiana Galochkina, Alexios Chatzigoulas, Zoe Cournia, Hubert Santuz, Sophie Sacquin-Mora, and Antoine Taly*
J. Chem. Inf. Model. 2024, 64, 1, 26–41
DOI: 10.1021/acs.jcim.3c01361
A Perspective on Protein Structure Prediction Using Quantum Computers
Hakan Doga*, Bryan Raubenolt*, Fabio Cumbo, Jayadev Joshi, Frank P. DiFilippo, Jun Qin, Daniel Blankenberg, and Omar Shehab
J. Chem. Theory Comput. 2024, 20, 9, 3359–3378
DOI: 10.1021/acs.jctc.4c00067
Unsupervisedly Prompting AlphaFold2 for Accurate Few-Shot Protein Structure Prediction
Jun Zhang*, Sirui Liu, Mengyun Chen, Haotian Chu, Min Wang, Zidong Wang, Jialiang Yu, Ningxi Ni, Fan Yu, Dechin Chen, Yi Isaac Yang, Boxin Xue, Lijiang Yang, Yuan Liu, and Yi Qin Gao*
J. Chem. Theory Comput. 2023, 19, 22, 8460–8471
DOI: 10.1021/acs.jctc.3c00528
AI-Based Protein Structure Prediction in Drug Discovery: Impacts and Challenges
Michael Schauperl* and Rajiah Aldrin Denny
J. Chem. Inf. Model. 2022, 62, 13, 3142–3156
DOI: 10.1021/acs.jcim.2c00026
Can Protein Structure Prediction Methods Capture Alternative Conformations of Membrane Transporters?
Tengyu Xie and Jing Huang*
J. Chem. Inf. Model. 2024, 64, 8, 3524–3536
DOI: 10.1021/acs.jcim.3c01936
Accurate Prediction of Enzyme Thermostabilization with Rosetta Using AlphaFold Ensembles
Francesca Peccati*, Sara Alunno-Rufini, and Gonzalo Jiménez-Osés*
J. Chem. Inf. Model. 2023, 63, 3, 898–909
DOI: 10.1021/acs.jcim.2c01083
AlphaFold, Artificial Intelligence (AI), and Allostery
Ruth Nussinov*, Mingzhen Zhang, Yonglan Liu, and Hyunbum Jang
J. Phys. Chem. B 2022, 126, 34, 6372–6383
DOI: 10.1021/acs.jpcb.2c04346
Enhancing Protein Function Prediction Performance by Utilizing AlphaFold-Predicted Protein Structures
Wenjian Ma, Shugang Zhang*, Zhen Li, Mingjian Jiang, Shuang Wang, Weigang Lu, Xiangpeng Bi, Huasen Jiang, Henggui Zhang, and Zhiqiang Wei
J. Chem. Inf. Model. 2022, 62, 17, 4008–4017
DOI: 10.1021/acs.jcim.2c00885
Allostery Illuminated: Harnessing AI and Machine Learning for Drug Discovery
Maria-Jesus Blanco*, Melissa J. Buskes, Rajiv G. Govindaraj, Jonathan J. Ipsaro, Joann E. Prescott-Roy, and Anil K. Padyana
ACS Med. Chem. Lett. 2024, 15, 9, 1449–1455
DOI: 10.1021/acsmedchemlett.4c00260
Inside-Out Design of Zinc-Binding Proteins with Non-Native Backbones
Sharon L. Guffy, Surya V. S. R. K. Pulavarti, Joseph Harrison, Drew Fleming, Thomas Szyperski, and Brian Kuhlman*
Biochemistry 2023, 62, 3, 770–781
DOI: 10.1021/acs.biochem.2c00595
Benchmarking Refined and Unrefined AlphaFold2 Structures for Hit Discovery
Yuqi Zhang, Marton Vass, Da Shi, Esam Abualrous, Jennifer M. Chambers, Nikita Chopra, Christopher Higgs, Koushik Kasavajhala, Hubert Li, Prajwal Nandekar, Hideyuki Sato, Edward B. Miller, Matthew P. Repasky, and Steven V. Jerome*
J. Chem. Inf. Model. 2023, 63, 6, 1656–1667
DOI: 10.1021/acs.jcim.2c01219
Improved cryoEM-Guided Iterative Molecular Dynamics–Rosetta Protein Structure Refinement Protocol for High Precision Protein Structure Prediction
Steffen Lindert* and J. Andrew McCammon
J. Chem. Theory Comput. 2015, 11, 3, 1337–1346
DOI: 10.1021/ct500995d
Prediction of protein conformation
Peter Y. Chou and Gerald D. Fasman
Biochemistry 1974, 13, 2, 222–245
DOI: 10.1021/bi00699a002
Explore additional ACS journal content surrounding the winning research topic:
Special Issues
AI for Synthetic Biology
ACS Synthetic Biology
Protein Engineering
Biochemistry
Computational Advances in Protein Engineering and Enzyme Design
The Journal of Physical Chemistry B