One way or the other, AI tools such as ChatGPT are determined to revolutionize our lives. But how close are we to an AI-powered assistant in the chemistry lab?
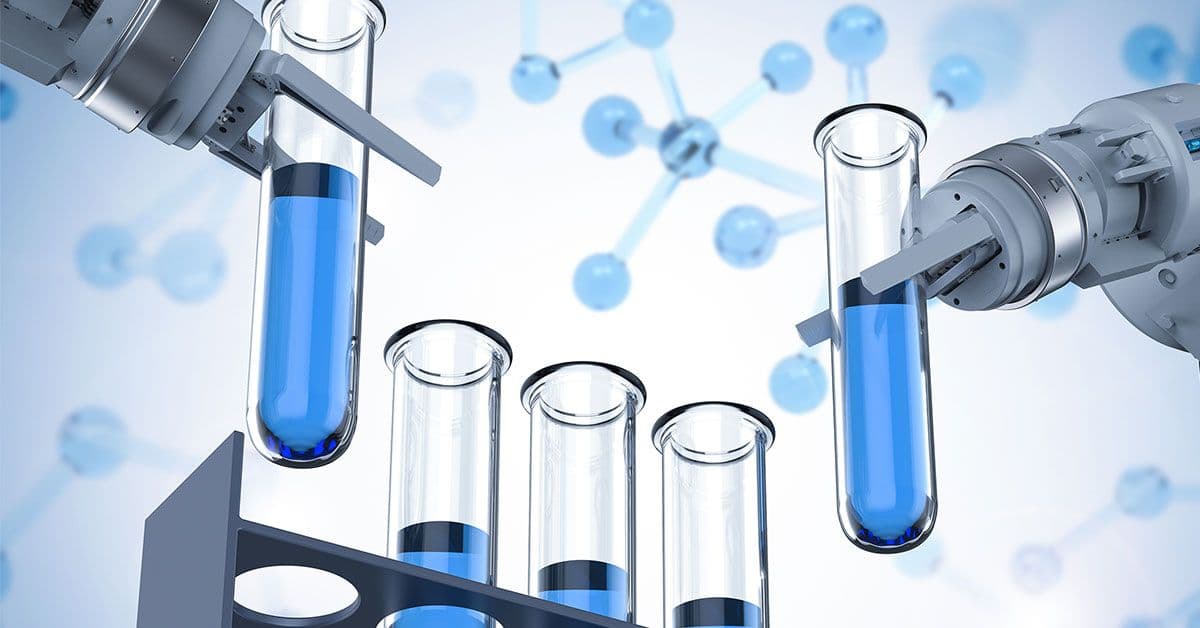
Generative pre-trained transformers (GPT) are a type of natural language generation models that can learn and fine-tune outputs based on prompts, response ranking, and proximal policy optimization. ChatGPT is the fastest-growing consumer application in history.
But beyond helping with school or work assignments—and writing amusing poetry—there are some attractive uses for this powerful technology. Consider the possibility of creating AI-powered chemistry assistants. If we can train AI in this way, it could revolutionize the chemistry landscape, allowing us to apply knowledge across disciplines and create efficiencies in tasks such as literature searches, compound screening, and data analysis.
Natural language processing (NLP) models have already been used in this way before. However, they are not always time-efficient, and they require expertise in coding and data science. NLP programs are also unable to generalize, so they must be rewritten when the target changes. With such gaps, tools such as ChatGPT could fundamentally transform the chemistry research process as we know it.
Early ChatGPT models appear to have a tendency to invent information—an unavoidable issue that makes relying on them in scientific fields challenging, to say the least. But a new study published in the Journal of the American Chemical Society suggests that prompt engineering could help get around this.
Researchers at the University of California, Berkeley, set out to train ChatGPT to text-mine metal–organic framework (MOF) synthesis conditions from scientific literature across a diverse range of formats and styles. Their approach uses a workflow with three different processes for text mining, programmed by ChatGPT itself. The authors report that these all enable parsing, searching, filtering, classification, summarization, and data unification with different trade-offs among labor, speed, and accuracy.
To test the system, the team extracted over 26,000 distinct synthesis parameters related to around 800 MOFs. Using the ChemPrompt Engineering strategy to instruct ChatGPT in text mining resulted in remarkable precision and recall performance.
With the resulting dataset, it was possible to construct a machine learning model which delivered over 87% accuracy in predicting MOF experimental crystallization outcomes. The authors were also able to create a MOF chatbot that could reliably answer questions about chemical reactions and synthesis procedures.
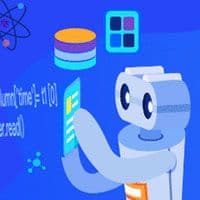
ChatGPT Chemistry Assistant for Text Mining and the Prediction of MOF Synthesis
DOI: 10.1021/jacs.3c05819
Similar work published in Chemistry of Materials describes the development of DigiMOF, a publicly available database for researchers that lets them rapidly search for MOFs with specific properties, analyze alternative production pathways, and create additional parsers to search for additional desirable properties. This is based on an adapted version of ChemDataExtractor (CDE), a chemistry-aware natural language processing tool.
What teams working with AI and MOFs seem to be agreeing on is that with their ability to quickly and rationally predict synthesis conditions, these tools can help accelerate the synthesis of new MOFs, bypassing traditional trial-and-error approaches.
These findings suggest that a ChatGPT Chemistry Assistant will be very useful across other chemistry subdisciplines as well. The technology builds on existing AI applications, which have enabled significant advancements in material discovery such as developing technologies for environmental research and sustainability. Other research has combined Monte Carlo tree searches and recurrent neural networks to discover MOFs specifically for carbon capture in humid conditions, demonstrating how AI can help push boundaries and uncover new knowledge.
However, as noted in a recent Chemistry of Materials editorial, we must keep in mind that while AI has proven to perform well within the spaces in which it is trained, its reliability often declines when pushed beyond that space. As such, chemists must still call the critical shots, guided by their own human expertise in the field.